Flow Cytometry Software, Reimagined
OMIQ is a modern cytometry analysis platform bridging machine learning and analytical pipelines with classical manual analysis.
How OMIQ Works
Combining high-performance analytical power and modern user-focused design
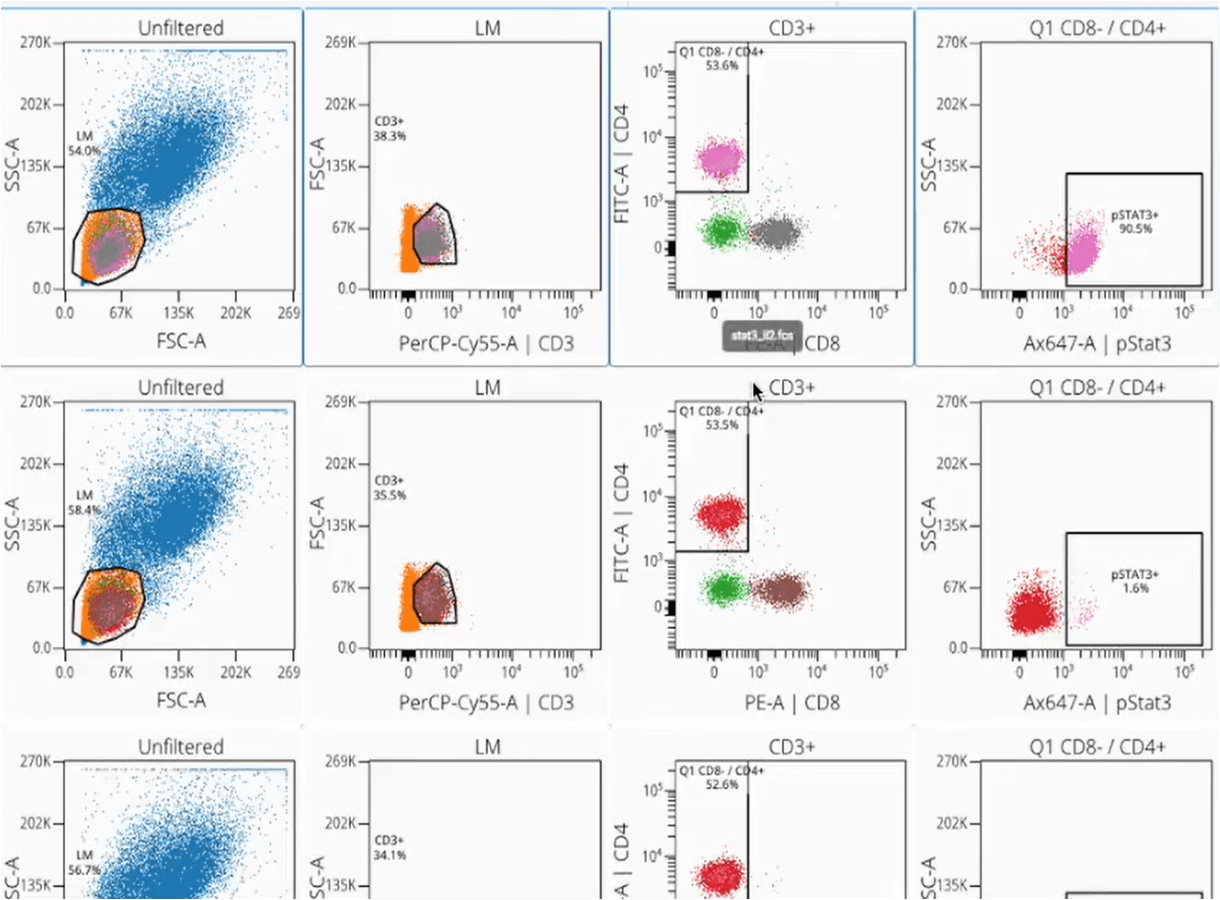
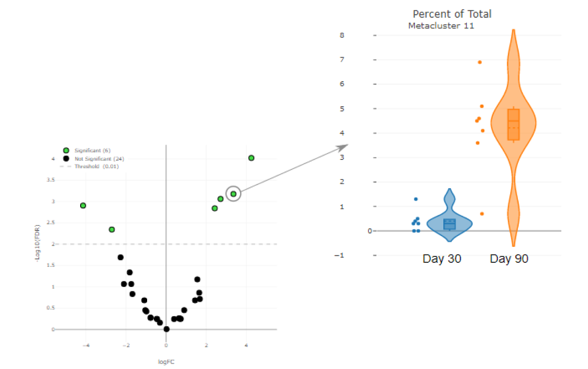
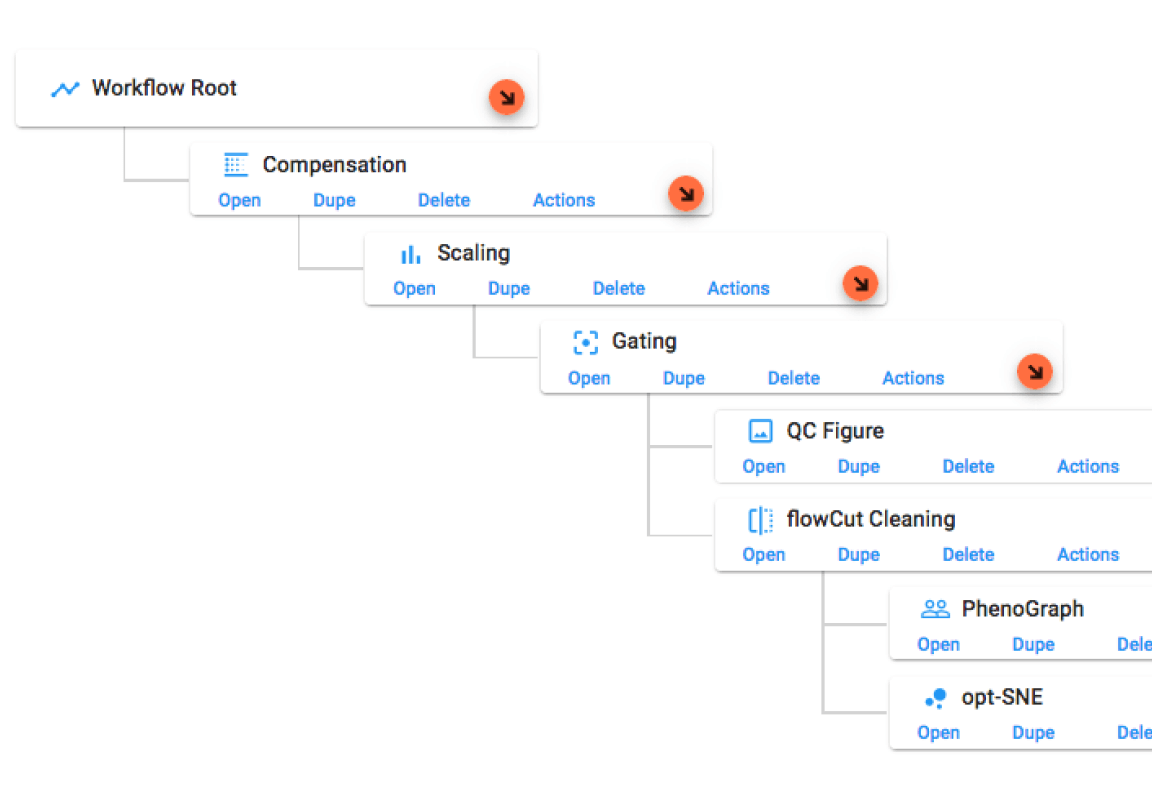
Trusted by the world’s leading research
organizations
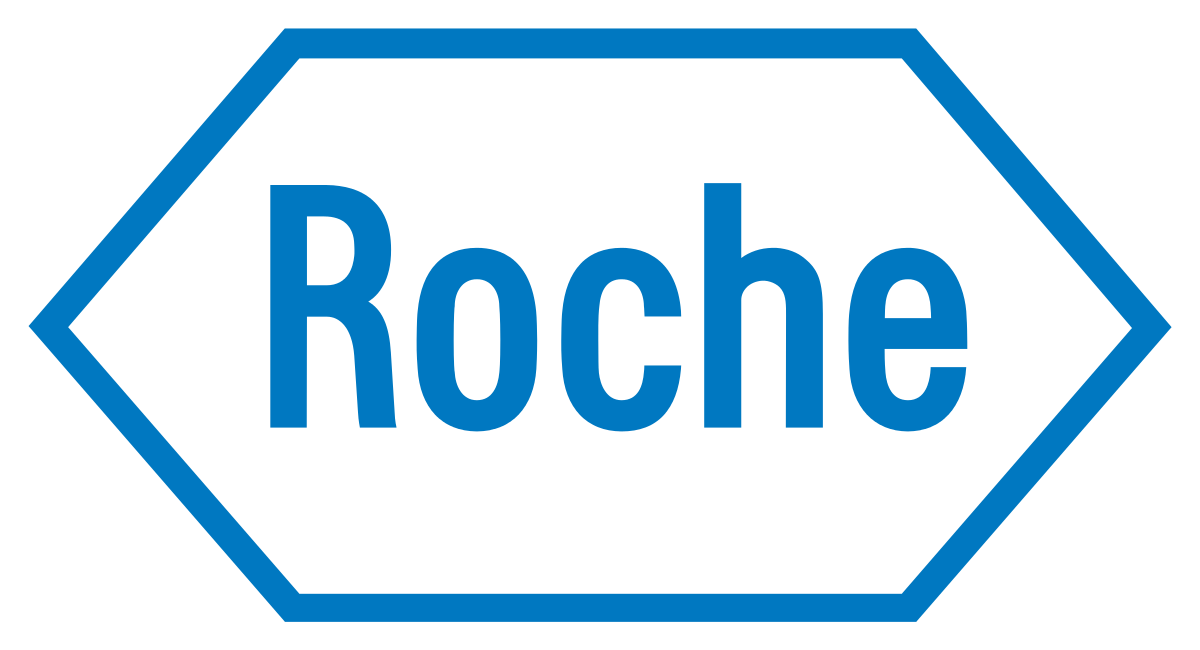
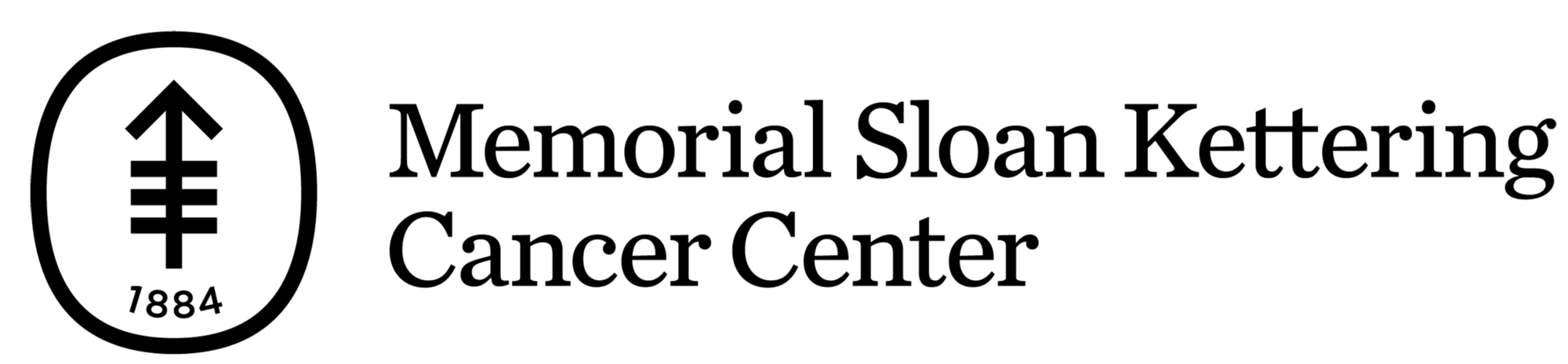
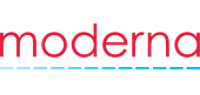
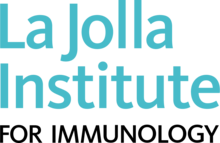
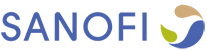
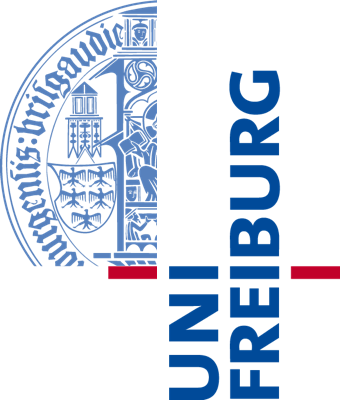
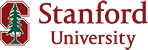

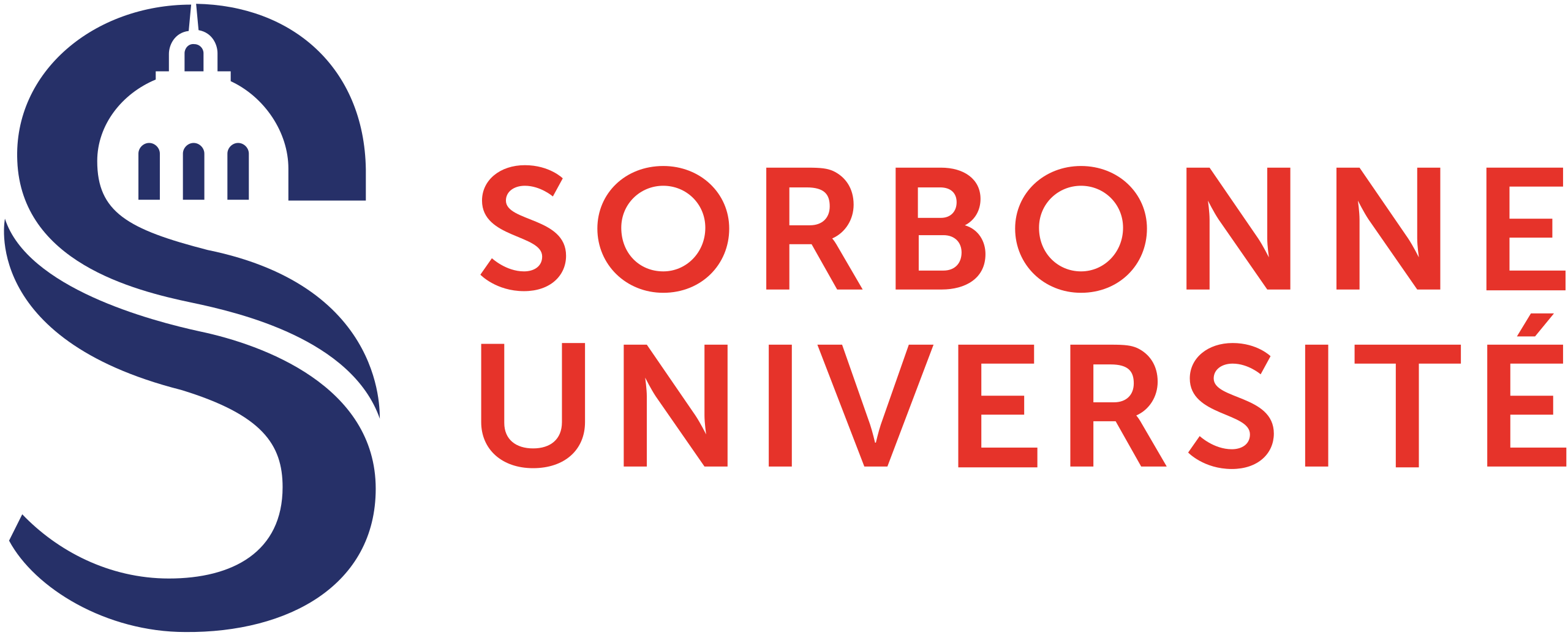

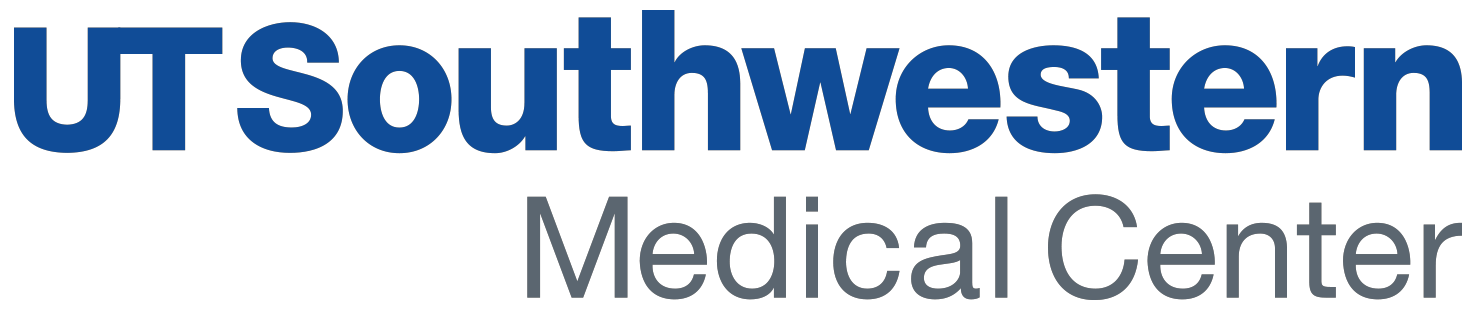


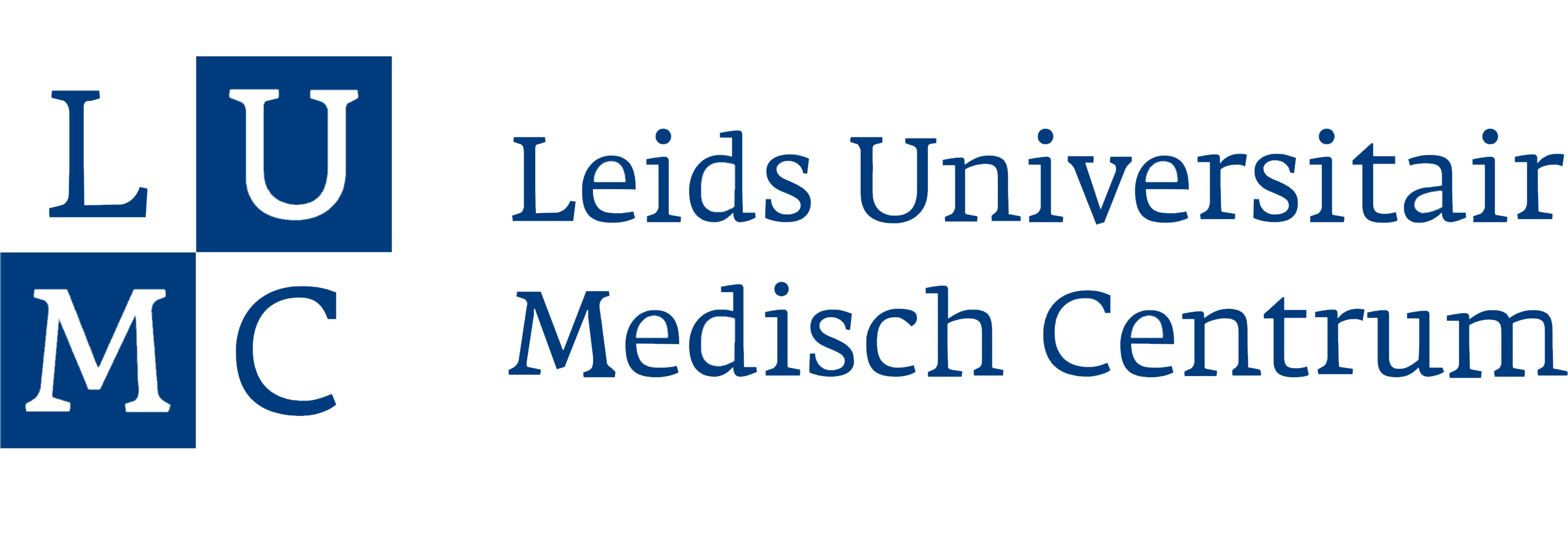
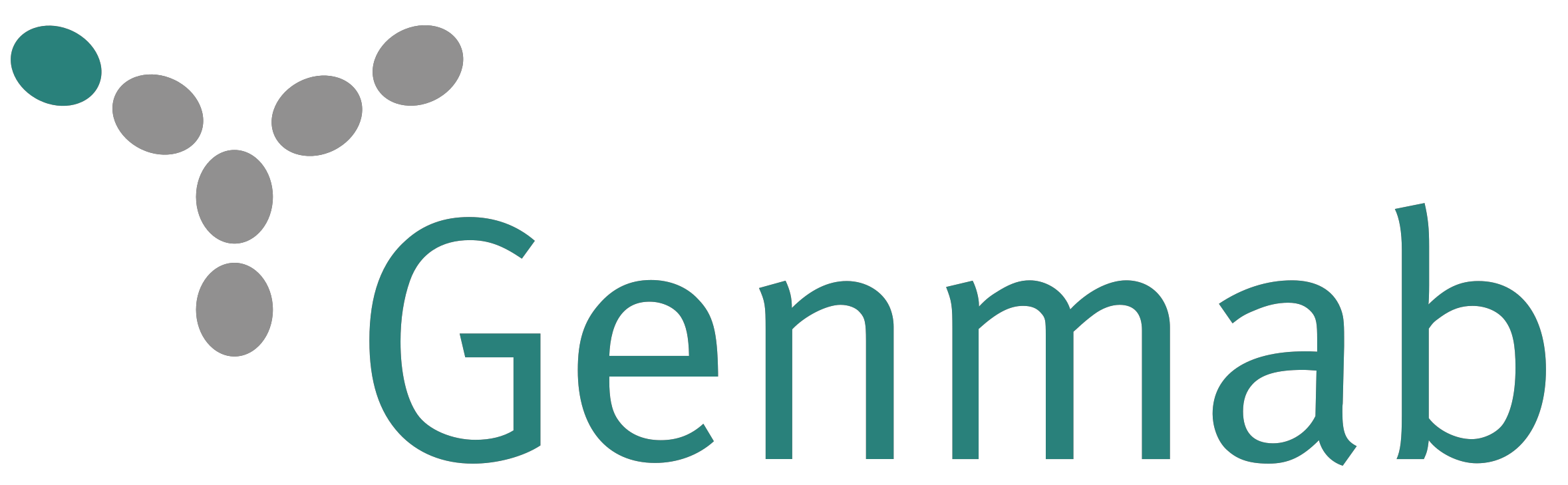

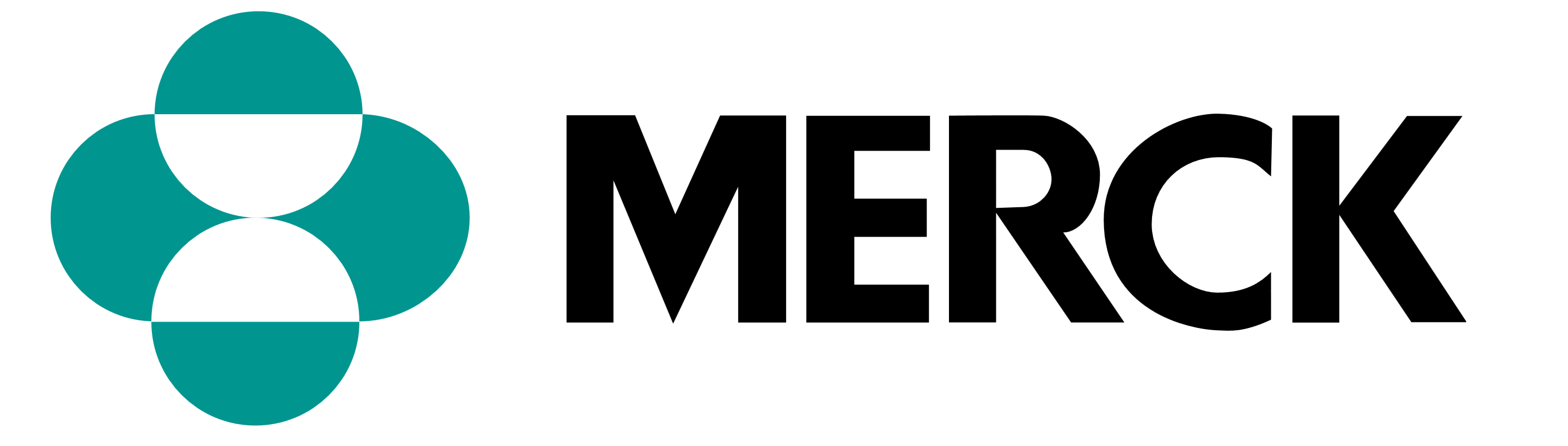
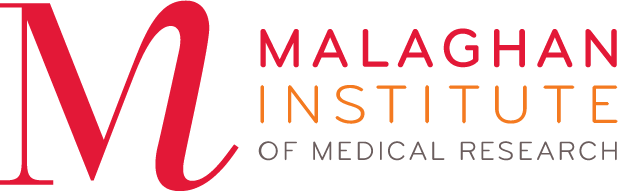
Experience the future of flow cytometry.